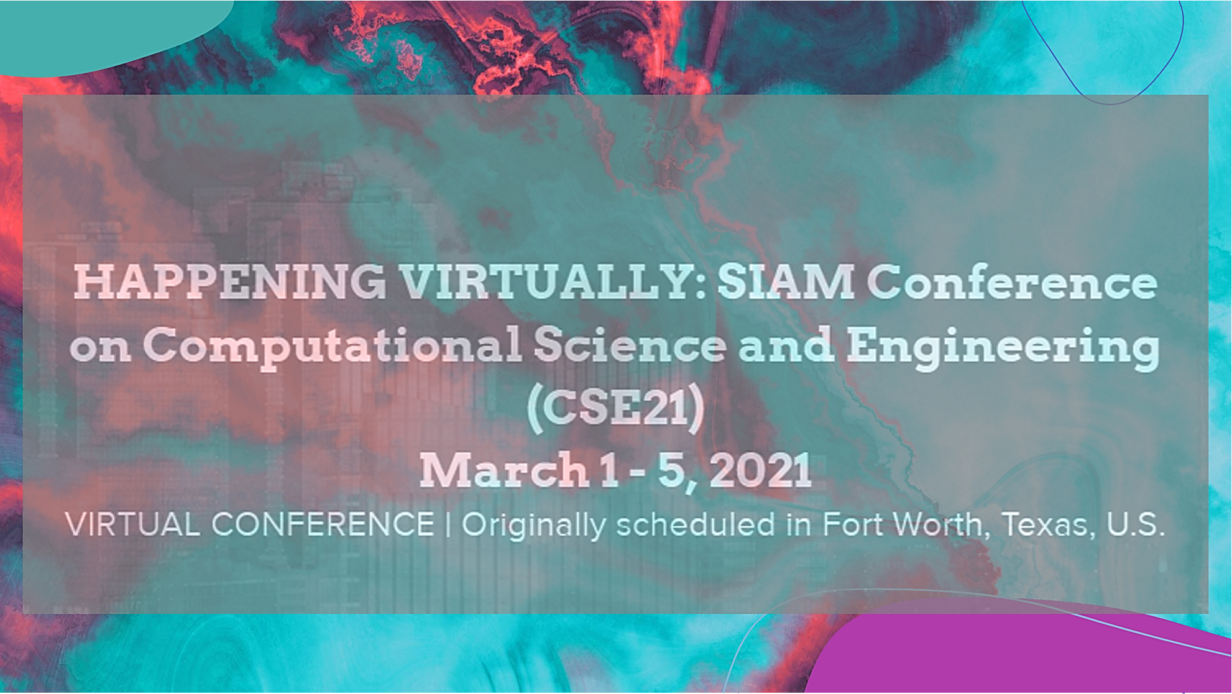
Science and engineering require reliable predictions. However, uncertainties affect every model and arise in every step of the computational process (model selection, parameter tuning, discretization and solver errors, likelihood of scenarios). In addition to this, reliability relates to accuracy, which frequently demands for computational time and resources. This motivates the interest for two synergic thrusts: (i) developing efficient computational strategies for resource-constrained settings, (ii) complementing the predictions with estimates of uncertainties and errors to provide decision makers with meaningful information. A great opportunity to address these challenges is to combine information from different sources (solvers and/or data) and with different levels of reliability in an effective way, typically by active/adaptive algorithms. Methods to this end lay at the intersection of computational science and machine learning with roots in applied statistics, uncertainty quantification, and approximation theory. In this thriving scenario, multifidelity modelling, adaptive sampling, Bayesian inversion, and data assimilation have been explored by many communities (sometimes under different names) and tailored to the specific use cases. The goal of the mini-symposium is to bring together researchers with different backgrounds to discuss the insights on these methods, their principled use in different domains, and their relevance to real-world applications. The mini-symposium is co-organized by CNR’s INM and IMATI together with Politecnico di Torino and MIT.
Mini-symposium info
MS300: Active Learning and Information Fusion for Reliable Predictions – Part I of II
MS331: Active Learning and Information Fusion for Reliable Predictions – Part II of II
Organizers
Laura Mainini
Politecnico di Torino, Italy and Massachusetts Institute of Technology, U.S.
Matteo Diez
CNR-INM, Italy
Lorenzo Tamellini
CNR-IMATI, Pavia, Italy
Program – Part I
- Active Learning and Information Fusion for Reliable Predictions: Session Overview
Laura Mainini, Politecnico di Torino, Italy and Massachusetts Institute of Technology, U.S.; Matteo Diez, CNR-INM, Italy; Lorenzo Tamellini, CNR-IMATI, Pavia, Italy - Optimization Approaches for Bayesian Inverse Problems
Claudia Schillings, Universitaet Mannheim, Germany; Bjoern Sprungk, Technical University Freiberg, Germany ; Philipp Wacker, Friedrich-Alexander-Universität Erlangen-Nürnberg, Germany - Surrogate-Based Adaptive Parameter Sampling for Interpolatory Model Order Reduction
Sridhar Chellappa and Lihong Feng, Max Planck Institute, Magdeburg, Germany; Valentin de la Rubia, Universidad Politécnica de Madrid, Spain; Peter Benner, Max Planck Institute for Dynamics of Complex Technical Systems, Germany - Resource Aware Active Learning for Multifidelity Optimization
Giorgio Manganini, Gran Sasso Science Institute, Italy; Francesco Grassi, United Technologies Research Center, Ireland; Michele Garraffa, University College Cork, Ireland; Laura Mainini, United Technologies Research Center, Ireland - Collective Decision Making for Sustainable Resource Exploitation
Vito Trianni, CNR, Italy; Dario Albani, CNR-ISTC, Italy
Program – Part II
- Multi-Information Source Optimization with Application to the Design of Materials
Doug Allaire, Texas A&M University, U.S. - Bayesian Regression for Aerodynamic Data Fusion Applications
Anna Bertram, German Aerospace Center (DLR), Germany - Data Fusion with Multifidelity Gaussian Processes for Aerodynamic Experimental and Numerical Databases
updated Andres F. Lopez-Lopera, Ecole des Mines de St Etienne, France; Nathalie Bartoli, Thierry Lefebvre, and Sylvain Mouton, ONERA, France; Joseph Morlier, Institut Supérieur de l’Aéronautique et de l’Espace, France - Physics-Based Machine Learning for Turbulent Flows and Forces
Gabriel Weymouth, University of Southampton, United Kingdom - Upscaling Solute Transport in Porous Media through a Trajectory-Based Spatial Markov Model
Giovanni Porta, Politecnico di Milano, Italy