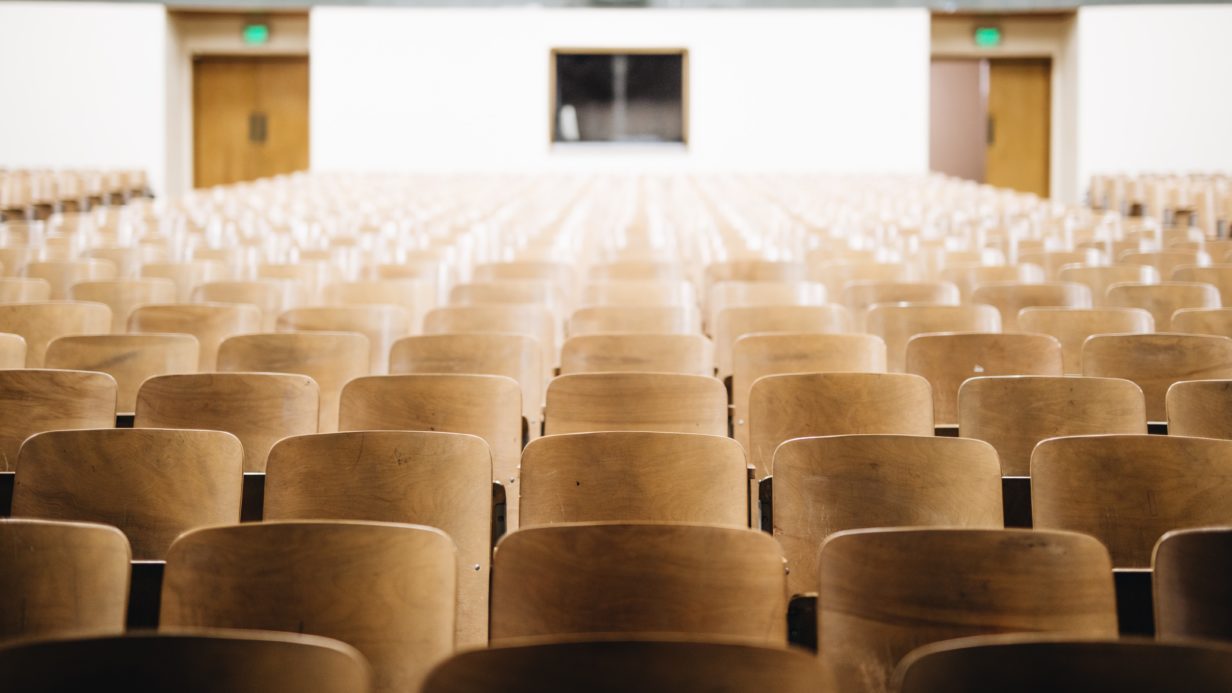
When and where
Thursday 17 October 2019, 12:00 Sala Consiglio, INM Headquarters Rome
Abstract
In many engineering applications governed by PDEs, the parameters of the equation (coefficients, forcing terms, boundary and initial conditions, shape of the domain) are not known exactly but rather affected by a certain degree of uncertainty, and can be described as random variables/random fields. Uncertainty Quantification (UQ) aims at estimating how the randomness of these parameters affects the “outputs” of the PDE, typically its solution or functionals thereof. UQ techniques are often based on repeatedly solving the PDE at hand for different combinations of the input parameters (i.e., a sampling approach), which requires a significant computational effort. To reduce such effort, so-called “multi-level” and “multi-index” methods have recently been proposed. These methods explore the variability of the PDE outputs using a hierarchy of suitably chosen discretizations, with the goal of exploring the variability of the outputs due to randomness on coarse discretizations at first, and refining the discretization only when needed; in other words, they aim at balancing the PDE discretization error and the sampling error. These methodologies are completely “black-box”, in the sense that they allow reuse of legacy PDE solvers, and are moreover trivial to parallelize. In this talk we describe in detail one such method, i.e., the so-called multi-index stochastic collocation (MISC) method, which is closely related to the quite popular sparse grids stochastic collocation method for the approximation of PDE with random data. In particular, MISC relies on PDE solvers with tensor structure. To this end, we use isogeometric analysis (IGA), which is a variation of the classical finite element method where the basis functions used by CAD designers to describe geometries (typically splines or related polynomials) are also used as a basis for the approximation of the solution of the PDE. We will discuss convergence results and show numerical results proving the effectiveness of the proposed method.
References: J. Beck, L. Tamellini, and R. Tempone. IGA-based Multi-Index Stochastic Collocation for random PDEs on arbitrary domains. Computer Methods in Applied Mechanics and Engineering, 2019.
Biography
Lorenzo Tamellini is a researcher at IMATI-CNR in Pavia. He was post-doc for three years at Ecole Polytechnique Federale de Lausanne; he got a PhD in Mathematical Models and Methods for Engineering in March 2012 at Politecnico di Milano and both Bachelor and Master Degree in Mathematical Engineering at Politecnico di Milano. His research activities focus on three topics: 1) Uncertainty Quantification and surrogate modeling for parametric PDEs 2) isogeometric analysis for PDEs 3) mathematical aspects of 3D-printing.