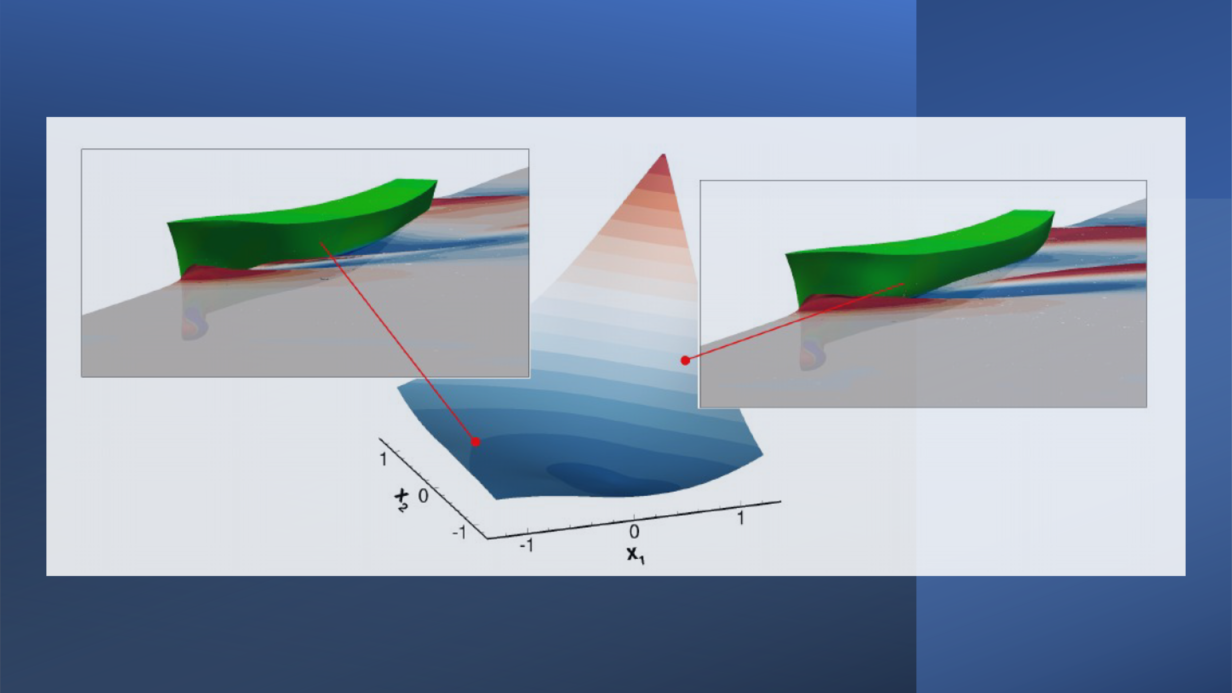
For the design of ships with low energy consumption or optimal performance in sports competitions, automatic shape optimization which uses numerical simulations in an automated search for the most efficient geometry, is a major new challenge. For shape optimisation using metamodels, the performances for a limited number of different geometries are evaluated thanks to numerical simulations. Then, an interpolation between these results gives an approximate model of the behavior of all the geometries, which is used for shape optimization. In a multi-fidelity setting, the efficiency of this metamodel is further increased by decomposing it into a low fidelity model, created from approximate but fast simulations, plus a correction from a few precise simulations.
Today, such metamodels are able to adapt to the simulation results on which they are based, estimate the noise in the simulations, and select automatically the designs for which new simulations must be performed [1]. On the other hand, many parameters, such as the fidelity levels and the total number of simulations, are still to be chosen by the user. However, the reliability and efficiency of the optimization process depend on these choices.
The objective of this project is to set up fully automated procedures to create multi-fidelity metamodels that are optimal for the behavior of the data on which they are based, as well as for the desired precision of the result. These procedures concern for example the elimination of simulation noise [2] and evaluating the contribution of each simulation fidelity to automatically choose the simulation fidelities to be retained. Such metamodels will use the available simulation power, optimally and without user intervention, in order to make automatic shape optimization affordable with the means available to a naval architect.
This work, which is funded by the institute Carnot MERS, is part of an ongoing collaboration between CNR-INM (Rome, Italy) and LHEEA on multi-fidelity metamodels [1,2] and also concerns a collaboration with IFREMER, IRDL and IRENav on the optimisation of lifting hydrofoils for sailing yachts (see for example [3]). The automatic simulation of hydrofoils, using the ISIS-CFD flow solver developed by Methric and included in FINE/Marine from Cadence Design Systems, is part of the current project. The optimisation of a realistic hydrofoil is one of the project objectives.
The post-doctoral researcher will be responsible for the creation, testing, and publication of the advanced multi-fidelity metamodels. The work will take place at Centrale Nantes with a number of working visits to CNR-INM. We are looking for a candidate with a Ph.D. in numerical mechanics, applied mathematics, or similar areas. (The Ph.D. has to be defended before the project start date.) Experience with numerical analysis and programming is required; knowledge of flow simulation, metamodelling, or shape optimisation would be appreciated. The capacity to work autonomously, the ability to work in a team, and good oral and written English communication skills are also required.
The official recruitment (https://www.ec-nantes.fr/english-version/recruitment) will start mid-ebruary 2022. Please contact Jeroen Wackers for any additional information on the project and the recruitment procedure (see announcement below).
References
- A. Serani et al. Adaptive multi-fidelity sampling for CFD-based optimization via radial basis function meta-
models. Int. J. Comp. Fluid Dyn. 33 (6-7), pp. 237-255 (2019). - J. Wackers et al. Multi-fidelity machine learning from adaptive- and multi-grid RANS simulations. 33rd Sym-
posium on Naval Hydrodynamics, online (2020). - M. Sacher et al. Flexible hydrofoil optimization for the 35th America’s Cup with constrained EGO method.
Ocean Eng. 157, pp. 62-72 (2018).